RamaOnHealthcare May 16, 2024
Building a Path Toward Precision Medicine with RWD & AI
Today, RamaOnHealthcare talks with Elliott Green, the Co-Founder and CEO of Dandelion Health. Dandelion Health is a real-world data and clinical AI platform powering next-generation precision medicine and personalized care. He combines in-depth understanding of the operational components of the U.S. payer, provider, and life sciences ecosystems with an ability to establish and manage complex institutional partnerships. He applies this experience within his latest AI-focused venture, Dandelion.
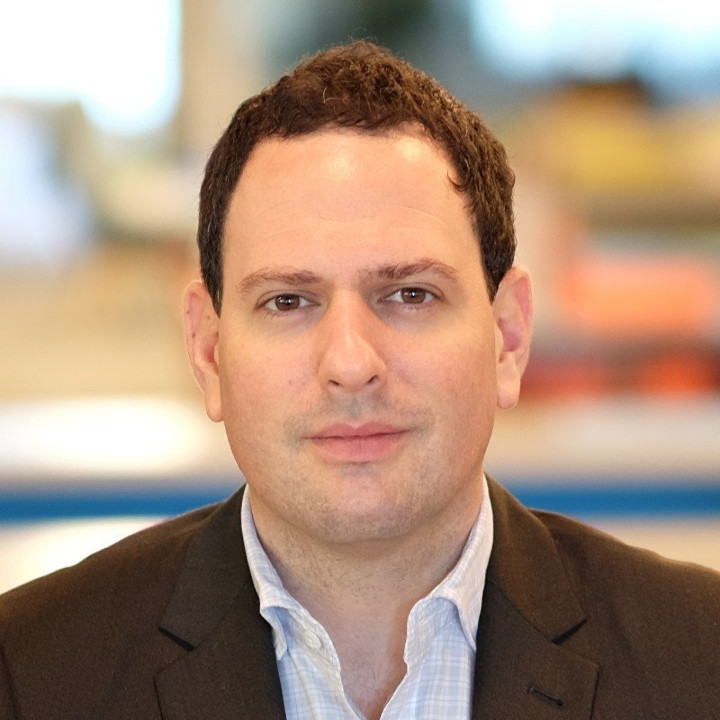
Elliott Green, Co-Founder and CEO of Dandelion Health
RamaOnHealthcare (ROH): As co-founder of Dandelion Health, can you tell us how the company came to be?
Elliott Green (EG): I am incredibly lucky to be surrounded by some of the brightest minds in AI and healthcare here at Dandelion Health (Dandelion). My co-founders, Sendhil Mullainathan, Niyum Gandhi, and Ziad Obermeyer and I all share one particular belief: Breaking down AI and data barriers in health and science holds the power to bring to life next-generation approaches to personalized care and precision medicine.
Having identified the need for technological advancement in healthcare, we often discussed what it would take for healthcare to embrace AI and machine learning (ML). A particular focus was how advanced technologies like AI and ML would “unlock” the power of siloed data — and not just the data contained in the electronic health record (EHR), but also broader sets of unstructured data housed outside of the EHR (i.e., waveforms, imaging data like CT scans, and more).
We collectively realized a life-improving AI/ML idea blossomed or withered almost always depended upon the quality and quantity of the clinical data that was used to build that AI/ML.
Our discussions naturally transitioned from ideation to business planning as we aligned on our vision here at Dandelion: To build the infrastructure that accelerates innovation in healthcare — improving clinical outcomes, lowering costs, and enhancing patient lives. To do that we needed to partner with forward-thinking provider organizations that had access to this data and shared these common goals. The creation of a consortium followed when we partnered with Sharp, Sanford, and Texas Health to create an ecosystem where data will drive meaningful positive change.
ROH: AI is all the rage in healthcare, and everywhere today. In healthcare, though, real trepidation remains. How is Dandelion, identifying unique ways to build trust in healthcare-specific AI technologies?
EG: Trust is one of the cornerstones Dandelion was founded on. In 2019, when we started Dandelion, our co-founders Ziad and Sendhil published a seminal paper in Science. They uncovered large-scale racial bias within a set of AI algorithms utilized across public and private healthcare settings. So, we started with a deep understanding of the potential risks and what it would take to address those and build safe and equitable AI.
We approach building trust in healthcare AI in five key ways:
Representativeness: To minimize bias in AI, the data used must accurately reflect the population the AI tools will serve. We have spent the past four years purposefully assembling a diverse consortium of healthcare systems from across the U.S. Together these systems represent data from millions of patients that enable findings to generalize across different patient groups, with the ability to understand race and ethnicity, urban or rural location, sex, and other social determinants of health.
We have spent the past four years purposefully assembling a diverse consortium of healthcare systems from across the U.S.
Quality: All the data in our platform is cleaned and harmonized, ensuring consistency across the systems. Because we centralize the data into one place, rather than use a federated model, the provenance of each data point (where it comes from) is also transparent. This allows researchers to see the raw data and ensure the data isn’t erroneous or violates key assumptions. We have exceptional documentation of our data transformations, ensuring we can trace data provenance right back to the raw data. We feel this is paramount to achieving accuracy.
Depth: We have access to all the patient data, including both structured data in the EHR and unstructured data such as clinician notes, images, and waveforms. We can go beyond the data captured by a claims dataset (which may be erroneous or missing diagnoses), providing a more enriched, accurate dataset.
Privacy and security: We prioritize privacy and security across every facet of our organization. To protect patients’ identities and privacy, we de-identify and tokenize data before it leaves the health systems’ environment while still maintaining the linkage of each individual patient’s data across their clinical journey. The data never leaves the Dandelion environment, and we make sure customers cannot download or make copies of the data.
We prioritize privacy and security across every facet of our organization.
Independent validation: Even with the above data to train AI algorithms, until we assess these algorithms independently, we cannot know how well they perform or whether they are biased against specific populations. This is why one of the first things we did as a company was to launch a free Validation Service. This enables any researcher with an AI algorithm to assess their algorithms for performance and bias on a large, representative, and independent dataset. We started with ECGs and chest CT algorithms. With the generous support from the Gordon and Betty Moore Foundation and The SCAN Foundation, and we look forward to expanding on these in the coming months.
ROH: How can industry better access and format unstructured data to improve data quality and the AI solutions built off that data?
EG: Many unstructured assets (images and waveforms) were never stored to be investigated as data for learning at scale. Instead, they were collated to be accessible to physicians looking to understand individual patient histories. Therefore, they are not easy to access or extract from their current home – such as servers in hospital systems. The amazing thing about this data is how high-quality it is. Unlike other information captured by human observation, this unstructured data represents raw biological information that provides incredible layers of understanding for AI. Our challenge is not to transform them, but simply to access them. This is what Dandelion’s health system partnerships are focused on.
ROH: You mentioned you’ll soon be launching a GLP-1 Data Library. Can you tell us how the data library will drive innovation for providers, researchers, and pharma?
EG: GLP-1s are set to be the biggest drug class of all time! We’ve already seen the promising impact of these drugs across obesity and diabetes and their potential to improve the lives of millions of patients. Yet the more these drugs make their way into routine healthcare, the more open questions we have regarding their impact. Are they effective for everyone? Are there potential unwanted side effects? Are there other potential benefits outside of obesity and diabetes?
Historically, answering these questions has taken decades and billions of dollars. If we wait on traditional clinical trials processes to answer these questions, we risk giving these GLP-1 drugs to patients without knowing the full downstream implications. At the same time, we may not be leveraging the potential of these drugs to their full capability to address other disease areas. Our team has spent the past year building one of the most comprehensive real-world datasets on GLP-1 usage and impact to help accelerate the answers to these questions — and to do so for a fraction of the time and cost of clinical trials.
Historically, answering these questions has taken decades and billions of dollars.
Our GLP-1 Library incorporates all available clinical data for millions of patients. What we saw when curating this dataset is what you tend to see across healthcare, more generally: The vast majority of the data is unstructured and locked away in clinical notes, images, waveforms, etc. — all of which are inaccessible to most researchers. But it is also critical to access to get a comprehensive view of GLP-1 usage and impact. By making the rich, multidimensional data offered by unstructured modalities available, accessible, and connected to real-world, treatment patterns and outcomes become available. The people who use the library can answer key questions about the critical role that GLP-1–based treatments play in clinical care.
Research-driven healthcare organizations such as life science companies, clinical research organizations, and others can use the GLP-1 Data Library for a range of use cases, including:
- Evaluating the quality of weight loss through endpoints found in body scans;
- Comparing the efficacy of treatments not studied head-to-head across a range of different real-world measures;
- Demonstrating GLP-1’s therapeutic effects beyond current FDA-approved uses, including secondary benefits derived from exploratory use or demonstrated with additional data modalities;
- Quantifying any side effects associated with GLP-1 use; and/or
- Developing precision-medicine tools to identify patients with uncontrolled symptoms or to match patients to the right treatments.
In short, with this GLP-1 Library, we are accelerating the move toward personalized medicine, which holds immense potential to transform healthcare.
…we are accelerating the move toward personalized medicine….
ROH: What is the most intriguing finding (or two) specific to weight loss drugs you’ve been able to identify thus far with your GLP-1 Library?
EG: There was an array of unique findings we’ve already been able to uncover through the Dandelion GLP-1 Library. For example, we found certain demographic groups saw greater weight loss over time. Older patients experienced more significant weight loss than younger people.
Another initial valuable finding from our GLP-1 Library is patients initiating GLP-1 therapies tend to have many other targetable comorbidities at baseline, which may or may not also be impacted using GLP-1s. For example: 30% have chronic kidney disease; 24% have obstructive sleep apnea; 15% have MASH or MAFLD; and 7% have heart failure.
ROH: Do you have any closing guidance for AI innovators in healthcare today? And how can AI developers specifically tap into the Dandelion platform — including the GLP-1 database to build more credible AI solutions?
EG: I appreciate the opportunity to share some tailored guidance for AI innovators focused on driving positive change in health and science. I have three focused pieces of advice:
- Know Thy Data: Not all health data platforms are the same. Seek out data partners that have led with intention and precision when it comes to gathering the most diverse, multimodal, and longitudinal healthcare data. It’s not about having the most data — it’s about having deep, raw data that ensures both transparency and trust when it comes to ensuring AI validation.
- Who You Partner with Matters: I use the word “partner” with purpose. It’s not hard to find an organization willing to sell you data, but it is incredibly challenging to find a data platform partner that will partner with you, hand-in-hand, to ensure trust and credibility are baked into the data that you are validating your AI algorithms on.
- Avoid “Mouse Traps”: Building with speed in mind is critical, but, more importantly, AI developers need to build with accuracy. Human lives and humanity are on the line, to some degree, when we talk about advances in healthcare-specific AI. And while regulations and guidelines are slowly surfacing from government and industry associations, it’s still a bit of the “wild west” out there for AI in healthcare and science. Don’t let the lack of guide rails today fool you into bypassing validation of your AI. This will, at some point come back to bite you when another AI developer comes to market with a similar tool that is fully vetted and validated.
- Finally, for AI developers looking to become part of the Dandelion ecosystem and/or specifically access our latest GLP-1 Data Library, please reach out to us here — we’d welcome the opportunity to collaborate.
…AI developers need to build with accuracy.
About Elliott Green
His career spans finance and healthcare technology, culminating in executive roles within healthtech startups that are focused on payers, providers, life sciences, and healthcare data. Also, Dandelion recently released a news announcement.
Health tech startup Dandelion Health launches data library to advance insights into GLP-1 drugs